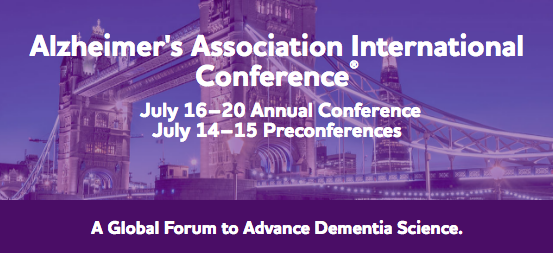
A new systems pathology paradigm of Alzheimer's disease
Background
Systems biology approaches to Alzheimer’s disease (AD) are comparatively rare [1] and reductionist concepts still prevail. We challenge the idea that either tau or Aß are the causal agents in the pathology of AD, and instead consider the role systemic factors play in its neurodegenerative spread through the brain.
Method
In a new systems pathology paradigm, we depart from a neuron-centric view of AD and instead expand it to consider the role of interactions between an ecosystem of cell types that play out in the complex spatial architecture in brain tissue. A mathematical model for the dynamics of AD is developed that draws together the latest results from molecular biology, pathology and imaging. The modelling approach incorporates data that explicitly describes the two-dimensional geometry of intracellular space. It also includes both the relative abundance and distribution of glial and neuronal cells, and their specific anatomical details such as the dendritic network. The mathematical equation set describes both intra and inter-cellular processes including signalling, cell movement, and transport of both tau and Aß in the intra and intercellular space.
Results
The model shows how characteristics of the spatio-temporal development of the disease can be understood in terms of the interactions between key elements, including tau and Aß, as part of an integrated system, as opposed to only considering these elements independently. More importantly, the resulting modelling framework constitutes a new systems pathology platform for AD that allows in silico experimentation to develop and test hypotheses, and will ultimately help integrate both accepted and developing theories from across the AD community.
Conclusions
We demonstrate the feasibility of tissue-level modelling of AD and suggest the potential of whole-brain modelling of AD. Our results suggest a shift in perspective from reductionist hypotheses about causal agents to systems-level thinking, may provide new insights into the processes that regulate the dynamics of the disease.
1Institute of Neurology, University College London, UK
2 Brain & Mind Centre, University of Sydney, Australia
3 Rothamsted Research, UK
[1] Rollo JL et al (2016). Unraveling the mechanistic complexity of Alzheimer’s disease through systems biology. Alzheimer’s & Dementia: The Journal of the Alzheimer’s Association.
A new systems pathology paradigm of Alzheimer’s disease
(AAIC 2017 Poster P4-062) – 2D simulations
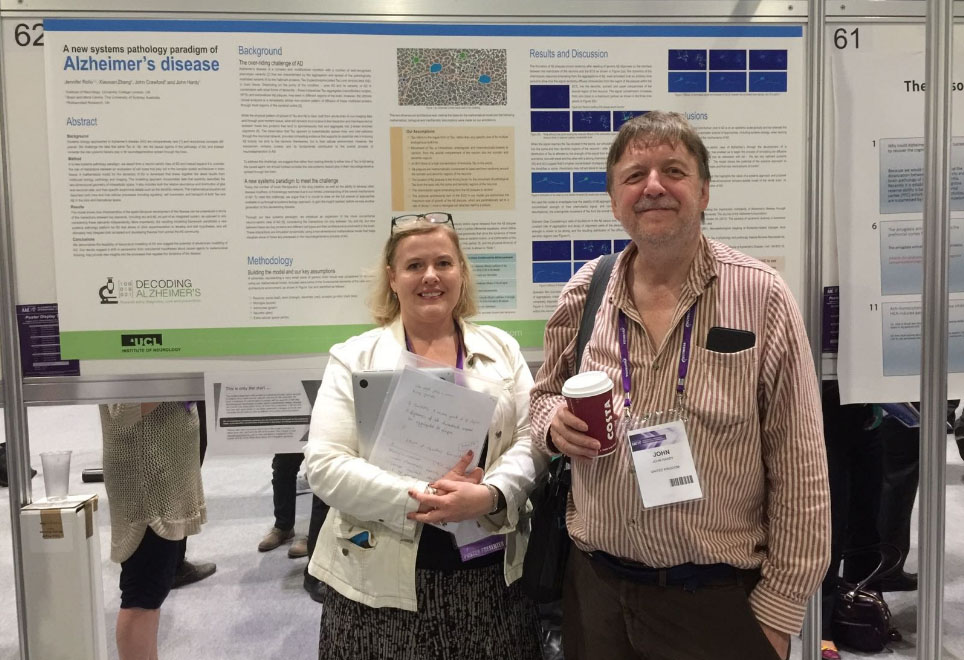
Me (Dr Jenny Rollo) and Prof. John Hardy in front of the poster summarising our pilot model at AAIC 2017.
The poster we presented at AAIC contained a number of screenshots from the following simulations that represent:
- Random seeding of Aß plaques
- Dynamics of the chemotactic signal from the Aß plaques
- Distribution of Tau in response to random movement
- Distribution of Tau in response to random movement and chemotaxis of varying strengths
- Diffusion of chemotactic signal from stable Aß plaques
- Diffusion of chemotactic signal from unstable Aß plaques.
The full simulations can be viewed below, and a jpg of the poster downloaded here or by clicking the button below the abstract to the left.
Simulating the random growth of Aß plaques
Formation of Aß plaques occurs randomly after seeding of generic Aß oligomers on the interface between the membrane of the cells and the extracellular space (ECS), plus some seeds are in the ECS itself around the soma and dendrites, not just on the interface.Two constraints imposed by the code:
- Aß can only aggregate in the ECS around the soma and dendrites of the neuron
- The maximum size of the Aß is controlled by a parameter in the code.
Simulating the distribution of Tau in response to random movement
Once the Aß plaques have aggregated on areas of the cell membrane/ECS interface, and also within the ECS region around the dendrites and soma of the two neurons, as shown above, we simulate the dynamics of the chemotactic response from these plaques.
The chemical signal released by the Aß plaques diffuses randomly intracellularly (within the axons, soma and dendrites of the neuron) and anywhere in the ECS.
The concentration of the signal increases from zero (blue) to a maximum (yellow).
For the simulations, we make the assumption that at time, t=0, there is a high concentration (yellow) of immobile hyperphosphorylated Tau located in the axon of the neuron. When the chemotactic signal diffusing from the Aß plaques reaches the Tau in the axon, Tau responds by starting to diffuse anterogradely into the soma and dendrites of the neuron. The movement of Tau can be modelled in one of two ways:
- Purely random
- Random with chemotaxis.
For both cases, the relative significance is controlled by a parameter in the code.
The three figures below show a snapshot of the relative effect of none, weak and strong chemotactic concentrations in combination with random motion of Tau. These results suggest that if a higher concentration of Aß plaques is located closer to the axon, rather than around the dendritic regions or the soma, chemotaxis may not act alone in causing the Tau’s movement anterogradely into the neuron body and dendrites.
Simulating the distribution of Tau in response to random movement and chemotaxis of varying strengths
Simulating the diffusion of chemotactic signal from stable Aß plaques
We used the model to investigate how the stability of Aß aggregates affects their chemotactic signal strength and consequently (based on our initial assumptions in the model), the movement of Tau from the axonal region of the neurons.
(a) Consider a state of equilibrium in the size of the Aß plaques, where size is controlled by a constant rate of renewal and decay of oligomeric parts of the Aß plaques.
It is not clear what determines the maximum size of the aggregates, but we are assuming in our model that the physical size of the ECS architecture in the region of each unique plaque, is the primary constraint of plaque size.
Simulating the diffusion of chemotactic signal from unstable Aß plaques
(b) Conversely, consider a higher rate of decay of soluble oligomeric parts of the Aß plaques to the rate of aggregation, so their size and therefore chemotactic signal strength is affected.
The model does not assume the plaques will completely degrade, but the level of degradation is higher than aggregation.
Comparing the two scenarios shows a clear dependence for the movement of Tau on the strength of chemotactic signal from the Aß plaques.
Both scenarios are controlled through parameters in the code.
Simulating the movement of microglia and signal from the Aß plaques
The following simulation is in addition to the information included on the static poster and demonstrates the movement of microglia and the responding chemotactic signal emanating from the Aß plaques. The model was set up with an arbitrary number of 20 microglia cells, with a weak repulsive force applied to each cell (controlled parametrically) to prevent them from becoming too close to each other. A stronger chemotactic signal, also controlled parametrically, was applied to the Aß plaques to attract the microglia.